Neuromorphic engineering, a field at the intersection of neuroscience and technology, is revolutionizing the way machines learn and interact with the world. By emulating the structure and function of the human brain, this innovative approach to computing enables the development of more efficient, adaptive, and intelligent robotic systems. This article delves into the key concepts and technologies that define neuromorphic engineering, tracing its historical evolution and exploring its transformative impact on robotics learning. From cutting-edge applications to the challenges and future directions of this rapidly advancing field, we will uncover how brain-inspired innovations are shaping the next generation of robotics.
uzocn.com will provide a detailed exploration of this topic.
1. Introduction to Neuromorphic Engineering
Neuromorphic engineering is an emerging discipline that bridges the gap between biology and artificial intelligence by replicating the architecture and processes of the human brain in electronic systems. Unlike traditional computing, which relies on sequential processing, neuromorphic systems operate in a parallel, event-driven manner, similar to the brain’s neural networks. This approach not only enhances computational efficiency but also enables machines to learn and adapt to new information in real time.
The field was born from the desire to overcome the limitations of conventional computing in tasks that require perception, learning, and decision-making. By mimicking the brain’s ability to process vast amounts of information simultaneously, neuromorphic engineering opens the door to more intelligent and responsive robotic systems. As researchers continue to refine these brain-inspired technologies, the potential applications extend far beyond robotics, promising to revolutionize industries ranging from healthcare to autonomous systems. Neuromorphic engineering is, therefore, a cornerstone of the future of artificial intelligence.
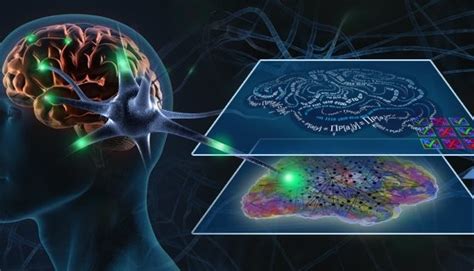
2. Key Concepts in Brain-Inspired Computing
Neuromorphic engineering, driven by brain-inspired computing, seeks to emulate the structure and function of the human brain. Key to this approach are spiking neural networks (SNNs), which depart from traditional artificial neural networks by transmitting information through spikes, mirroring the way neurons communicate through electrical impulses. This spike-based processing enables more dynamic and efficient data handling, closely mirroring the brain’s capacity for real-time adaptation.
Synaptic plasticity, a fundamental principle of the brain, enables the strengthening or weakening of synapses through learning and experience. This concept is mirrored in neuromorphic systems, where circuits possess adaptability and self-learning capabilities, evolving as they encounter novel information. These systems also employ event-driven processing, executing computations only when necessary, thereby minimizing energy consumption and maximizing efficiency.
By incorporating these principles, brain-inspired computing aspires to develop machines capable of handling information in a more natural and intuitive manner, thereby laying the groundwork for cutting-edge applications in robotics and other domains.
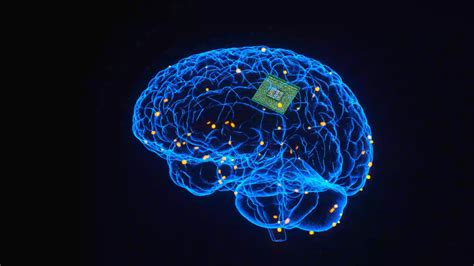
3. Historical Development and Evolution
The roots of neuromorphic engineering can be traced back to the 1980s, a time when pioneers like Carver Mead began to recognize the shortcomings of traditional computing in emulating the intricate workings of the human brain. Mead, a leading figure in this field, envisioned the creation of electronic circuits that would mirror the neural structure of the brain, thus laying the foundation for neuromorphic engineering.
In the early stages, the focus was on creating simple analog circuits that could emulate basic neural functions. Over time, advances in semiconductor technology enabled the development of more sophisticated neuromorphic chips, capable of handling increasingly complex tasks. The introduction of spiking neural networks (SNNs) in the 1990s further propelled the field, allowing for more accurate modeling of neural processes.
As computational neuroscience and machine learning evolved, so did neuromorphic engineering, leading to its current role in advancing robotics and artificial intelligence. Today, neuromorphic systems are at the forefront of research, offering promising solutions for real-time, adaptive, and energy-efficient computing.
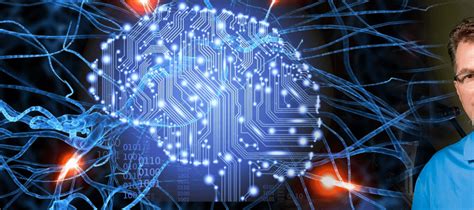
4. Core Technologies and Components
The foundation of neuromorphic engineering lies in several core technologies and components that enable brain-inspired computing. At the forefront are neuromorphic chips, specialized processors designed to emulate the neural architecture of the brain. These chips, such as IBM’s TrueNorth and Intel’s Loihi, feature millions of artificial neurons and synapses, enabling them to process information in parallel and respond dynamically to environmental stimuli.
Spiking neural networks (SNNs) are another critical component, operating in a way that mirrors the brain’s use of electrical impulses to transmit information. These networks allow neuromorphic systems to process data in an event-driven manner, significantly reducing energy consumption and improving efficiency compared to traditional processors.
Synaptic transistors are also pivotal, enabling the implementation of synaptic plasticity in hardware. These devices adjust their conductivity based on previous activity, mimicking the learning and memory functions of biological synapses.
Additionally, the development of memristors, resistors with memory capabilities, has advanced the creation of non-volatile memory systems that retain information without power. Combined, these technologies form the backbone of neuromorphic engineering, driving innovations in robotics and paving the way for more intelligent and adaptive machines.
5. Applications in Robotics Learning
Neuromorphic engineering is revolutionizing robotics by empowering machines to learn and adapt in ways strikingly similar to human cognition. A key application in this field is the creation of autonomous robots capable of processing sensory data and making real-time decisions. By utilizing spiking neural networks and neuromorphic chips, these robots can interpret intricate environments, identify patterns, and react to dynamic changes with unprecedented speed and precision compared to conventional systems.
Neuromorphic systems empower robots to execute tasks like object recognition, motion planning, and navigation while requiring minimal computational resources. Moreover, these systems enable reinforcement learning, allowing robots to enhance their performance progressively through trial and error, mirroring the way humans acquire knowledge from experience.
Neuromorphic technologies are revolutionizing collaborative robotics by enabling robots to seamlessly interact with humans. By understanding and predicting human behavior, these technologies empower robots to be more intelligent, adaptable, and efficient in a wide range of real-world applications, pushing the boundaries of what robots can accomplish.
6. Recent Advances and Breakthroughs
Neuromorphic engineering has made significant strides, yielding breakthroughs in both technology and applications, particularly in robotics and artificial intelligence. A key development is the advancement of neuromorphic chips, exemplified by Intel’s Loihi 2 and IBM’s latest TrueNorth iterations. These chips boast enhanced processing power, greater energy efficiency, and improved scalability. This progress has enabled the integration of more complex spiking neural networks into robotics systems, empowering them to tackle increasingly intricate tasks.
Flexible neuromorphic materials are another significant development, paving the way for more adaptable and durable robotic systems. These materials enable the creation of sensory skins and prosthetics that emulate the tactile sensitivity of human skin, thereby enhancing the interaction between robots and their surroundings.
Neuromorphic principles are rapidly being applied in edge computing, empowering robots to process information locally, rather than relying on cloud-based systems. This localized processing minimizes latency and energy consumption, resulting in more efficient and responsive neuromorphic robots. These advancements are laying the groundwork for the development of future intelligent, adaptive, and energy-efficient robotic systems.
uzocn.com